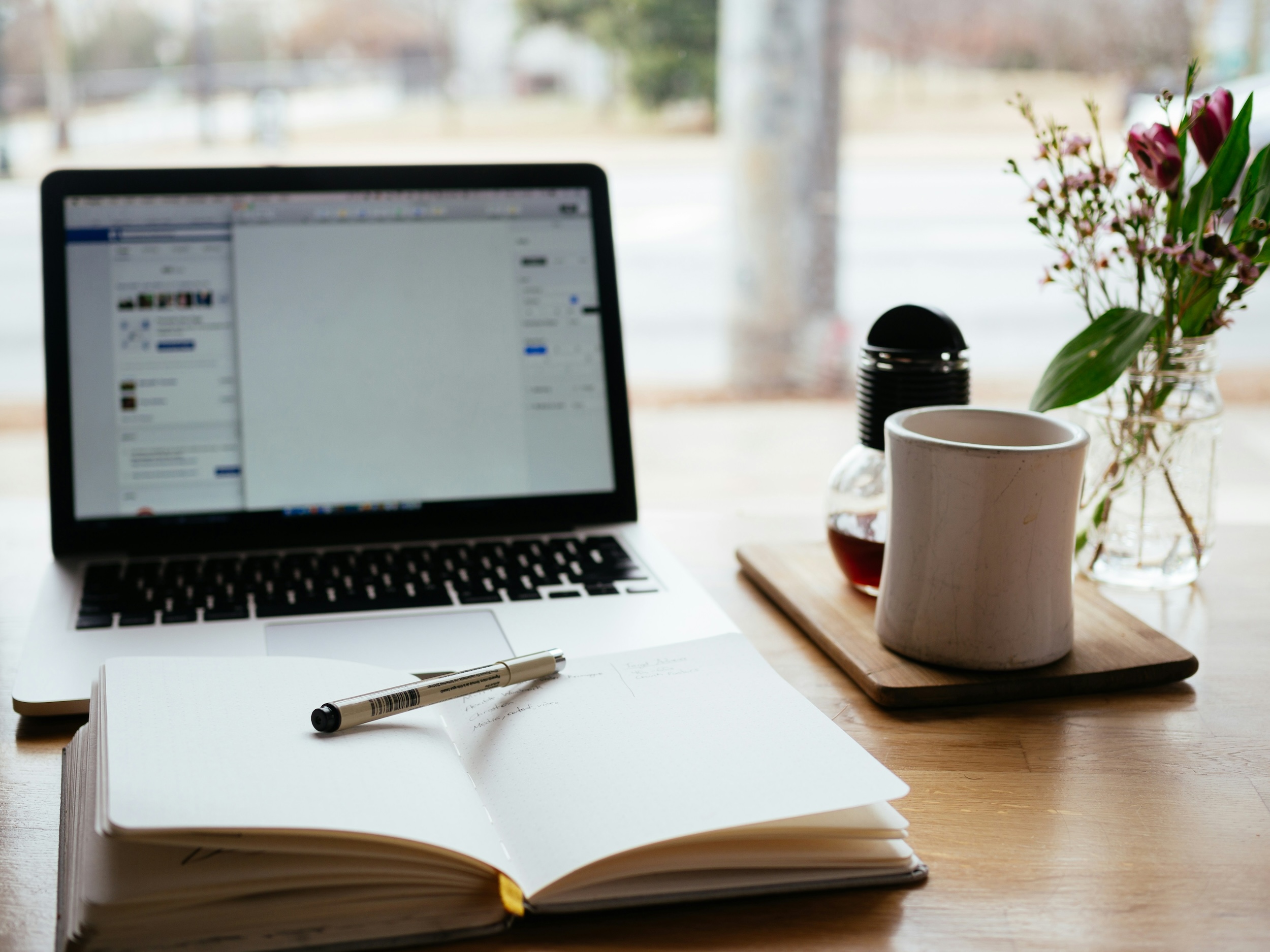
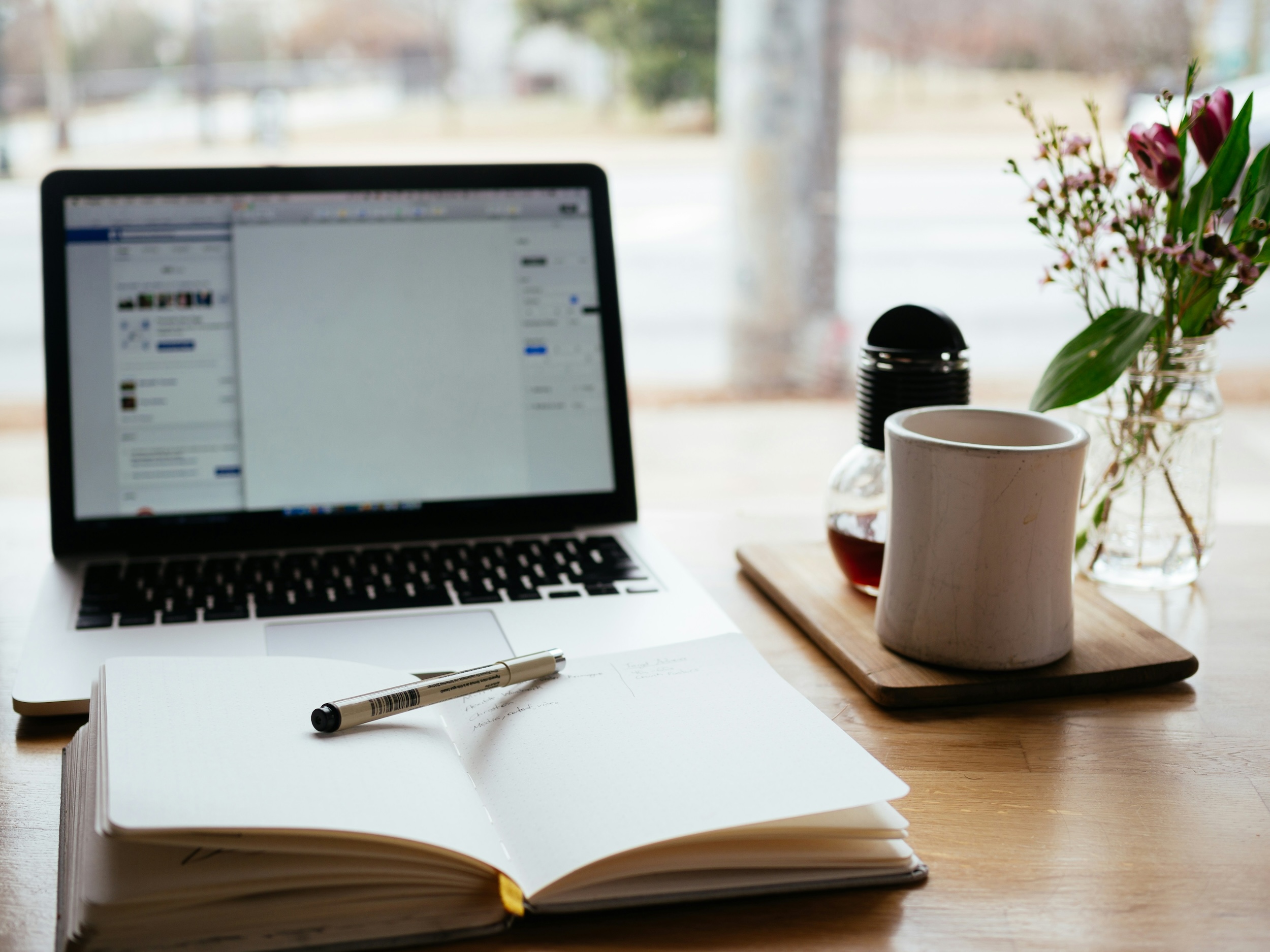
Adaptive or personalized learning is a promising concept, providing the learner with a tailored learning experience at any time. Despite the increasing interest of learning designers in adaptive learning, the lack of clear definitions and structured theoretical models has led to inconsistent implementations. Jan L. Plass & Shashank Pawar (2020) offer precise definitions of key terms related to adaptivity and personalization. They also describe different types of adaptivity in the form of a taxonomy to guide designers and researchers of adaptivity systems.
The authors first define several key terms to specify the concept of adaptivity:
Adaptive systems should consider cognitive, motivational, affective, and socio-cultural factors to optimize learning outcomes. Designers should evaluate these variables’ relevance, variability, and knowledge to justify adaptivity.
Effective measurement of learner variables is crucial for guiding adaptation. Methods include pre/post-tests, real-time tracking, and advanced analytics like educational data mining and learning analytics.
Adapting the Learning Environment
Adaptation can be approached through:
Plass and Pawar categorize adaptivity into macro-level (broad adjustments over time) and micro-level (real-time adjustments during learning) adaptations. Emerging forms of adaptivity leverage AI and technology for expanded personalization.
Challenges and Future Research
Adaptive learning faces challenges such as a lack of empirical research on non-cognitive adaptivity, ethical and privacy concerns, and resource-intensive implementation. Further research is needed to refine adaptive models for scalability, effectiveness, and ethical soundness.